Eli Review co-creator Jeff Grabill delivered they keynote address for the 2016 meeting of the Computers & Writing conference on Saturday, May 20, 2016 in Rochester, New York. His talk, titled “Do We Learn Best Together or Alone? Your Life with Robots”, discussed learning technologies and robots, particularly what they are and aren’t good for. He also discusses what role robots have in the classroom and the power that teachers have to shape technologies and technology policy on their campuses.
The full video of Jeff’s keynote is embedded below, followed by the full transcript and reactions from attendees. Note: this video was originally livestreamed on Eli Review’s Facebook page, where it can still be found and shared.
Opening: On Robots
In 2011, I helped Bill Hart-Davidson and Mike McLeod write a short piece on what we saw as important for the near future in computers and writing.
Our answer was one word: Robots.
We wrote something like this:
We believe we are already seeing the beginning of the next wave of technologies that will bring about large-scale changes in how written communication is practiced and how it is valued. It will not be a single lynchpin technology like the printing press nor will it be a platform like the Internet. It won’t even be a protocol. It will be a class of small (or small-ish) automated analytic functions assigned to do tasks that are incredibly tedious, repetitive, distributed in space (and perhaps time). This will be a new class of “little machines” driven by logic but put to work for explicitly rhetorical purposes. (doi:10.1016/j.compcom.2011.09.004)
Robots.
We argued for why robots can write and named a few of the ways that robots will be—and certainly presently are—engaged in many writing practices, augmenting and extending human capability across what we have accepted for thousands of years to be the range of rhetorical performance: invention, arrangement, style, memory, and delivery.
We encouraged our audience not to fear Robots, but rather build robots and otherwise engage deeply with the many issues that robots raise for how we write and learn to write.
It’s now 2016, and I can confidently report that identifying robots in relation to the future of writing can no longer be seen as controversial or particularly insightful.
I can also report that lots of people have been paying attention to robots and making them. There are a great many robots designed to write and to teach writing.
But I can’t report that writing teachers or writing experts or very many of us in this room have engaged.
We have left this important work to others. We’ve been interested in other things.
We’ve made a big mistake, and in my happy-go-lucky talk tonight, I will try to unpack that mistake. What was opportunity in 2010 is a challenge at the present moment.

Part 1: The Chomsky-Norvig debate on AI and Science
In this first part, I think it’s important to dip our big toes into particular forms of machine intelligence because in referring to robots tonight, I’m really referring to the application of machine learning techniques to learning writing.
I focus on what became known as the Chomsky-Norvig debate, an exchange that started at MIT at about the time we wrote about Robots during a celebration of work on artificial intelligence. Chomsky, of course, is arguably the most important linguist of the 20th century whose work is foundational to many machine learning approaches. Peter Norvig is one of the planet’s foremost experts on artificial intelligence who is just as well-known for leading the research operation at Google. And I should state clearly at the outset that Les Perelman has been my guide here, pointing me to this debate as important and talking to me about his own current work that speaks to the issues that I will lay out here.
Here is a simple take on the debate.
Chomsky still wishes for an elegant theory of intelligence and language that helps us understand thinking and language. Chomsky argues that only models help us advance science and human understanding.
Norvig is said to believe in truth by statistics. The chase for models is slow and probably misguided. With enough data, the thinking goes, we can solve real problem without modeling. With, instead, what Norvig calls an “algorithmic modeling culture.”
With regard to language, what we might call “the Google approach” is to use increasingly advanced statistical reasoning and techniques to produce real solutions to, say, stylistic analyses of writing and machine provided feedback to students about writing quality.
The goal is not to develop a more sophisticated understanding of language. It is to try to get more data and build more powerful analytical tools.
All of this is directly relevant to writing and the teaching of writing, but there are no clear good guys and bad guys in this debate.
Having said that, however, and inspired by Perelman still, I am going to briefly lay out a problem that takes up the position of Chomsky (and Perelman).
OK, so Chomsky “wishes for an elegant theory of intelligence and language that looks past human fallibility to try to see simple structure underneath.” An explanatory theoretical model that enables science.
And so, in Chomsky’s own words, we get this:

“In my own field, language fields, it’s all over the place. Like computational cognitive science applied to language, the concept of success that’s used is virtually always this. So if you get more and more data, and better and better statistics, you can get a better and better approximation to some immense corpus of text, like everything in The Wall Street Journal archives — but you learn nothing about the language.”
You learn nothing about the language.
The analogy here—and I am confident that this is fundamentally Perelman’s argument, is that machine intelligence as applied to writing gives new and genuinely interesting patterns and even predictions but we learn very little about writing.
Let me go over this again. The whole point of learning technologies in a writing classroom is to help human beings, teachers as well as students, learn something about writing. Period.
If the machine doesn’t facilitate learning, then it isn’t useful. It might be smart, amazing, lovely. But it’s fundamentally useless.
What is worse—and I know that Perelman worries about this a great deal—the machines might actually do harm.
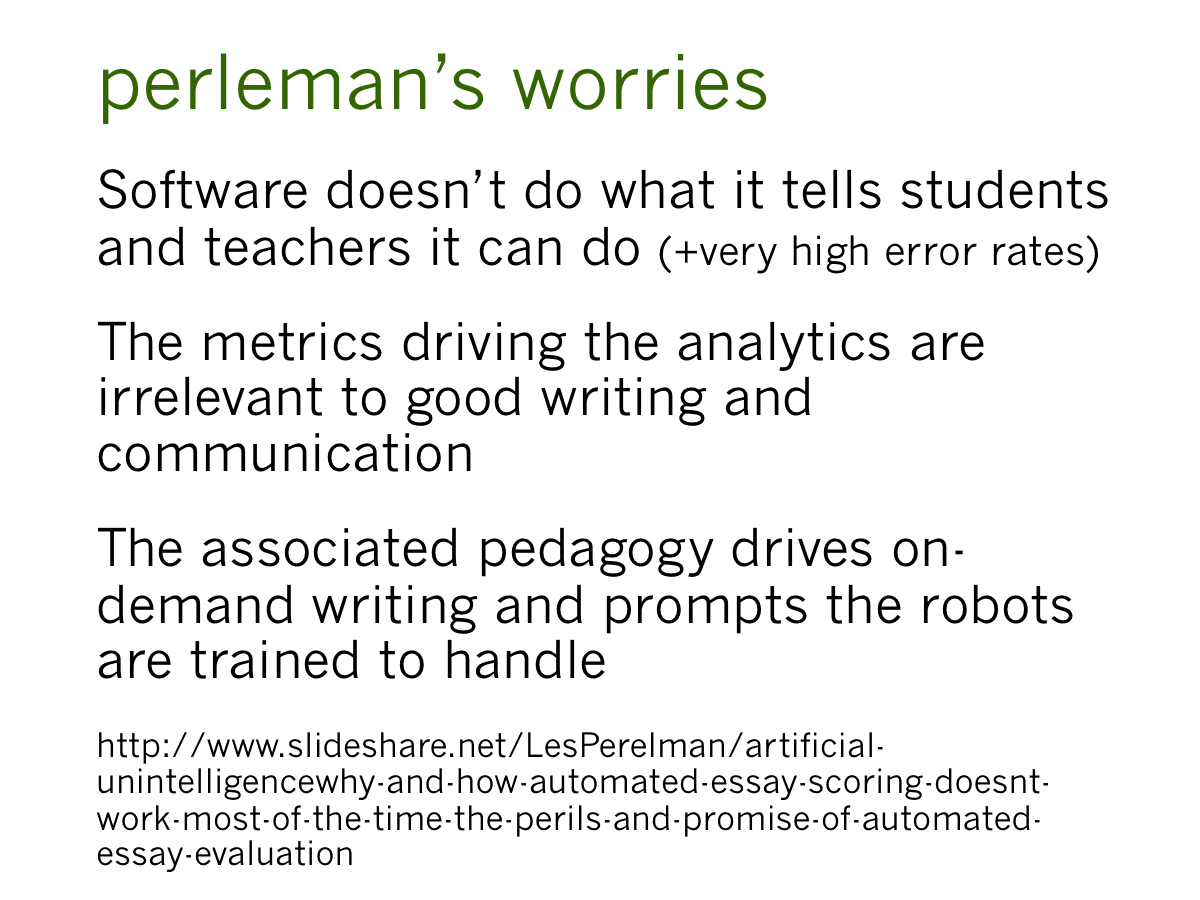
We can feel good, smug even, about the concerns and critique I’ve summarized here. We know better, we think. We don’t often dabble in these sorts of machine-driven pedagogies and practices.
Maybe.
Or maybe we are just irrelevant. In the marketplace right now, there are at least 8 serious products that promise to improve writing via some sort of robot. And there are many more robots running around out there embedded in other things. Almost none of them were developed by teams with anything close to a fraction of the writing expertise assembled in this room.
If we look at some of the most interesting research on peer learning, research that is located in the domain of writing instruction for all sorts of interesting reasons, none of the researchers are writing researchers.
Our absence is not simply a problem. It’s a disaster. Not simply for teachers and students but for this nice world we live in composed of humanist writing and technology experts.
Let me put a fine point on it and be argumentative: we should know better. We have chosen to be irrelevant because we haven’t, as a group, engaged the necessary research and development work to be relevant. The work that others are doing with technologies for learning to write has the potential to impact millions of learners.
Part 2: A Short Interlude on Robots Or: The Difference Between Educational Technologies and Learning Technologies
In 2010, we probably thought that this is what we had in mind about robots, and it might be what you have in mind for robots.

But as you can probably figure out, the reality of robots is more like this.

Those of you with a memory of Shoshana Zuboff’s work from ages ago on the difference between automating and informating technologies will see her work in this table.
One of the things we’ve been working with at Michigan State and also with Eli are the differences between educational technologies (or technologies for) and learning technologies (or technologies with).
I will tell you that one of the privileges of being an associate provost for teaching learning and technologies is that I can suggest that my campus adopts this heuristic thinking with regard to our learning technology investments. The table is an important distinction and I want to suggest to you that you can apply this heuristic to a lot of technologies that are on your campus and a lot of the technologies in the marketplace. It’s important to me and it comes out of the work I’ve been doing for the last three years with high school teachers in the state of Michigan because they’re at the front lines of this, much more so than we are.
Technologies for automate teacher work. If we had more time I’d tell you about me decade of experience in the venture capital world where they are out to replace you. They replace the teacher focus on summative testing, summative feedback, deprofessionalize teacher work, and they are free.
I want to dwell for a minute on free. Academic humanists might be the last people in the world who believe there is something called free. There is no such thing as free. You’re paying for your technology – you might be paying for it by making your students give up their personal data, or by giving up your own data, or you may be giving up technical or learning support. But you’re paying for it, and one of the most insidious moves in educational technology is schools penchant for free on the surface, which costs them dearly downstream, particularly in the toll it takes on the lives of teachers. There is absolutely no such thing as free, and when you’re confronted with free, you’re confronted with the nasty robot, and you should be asking very hard questions of people peddling free.
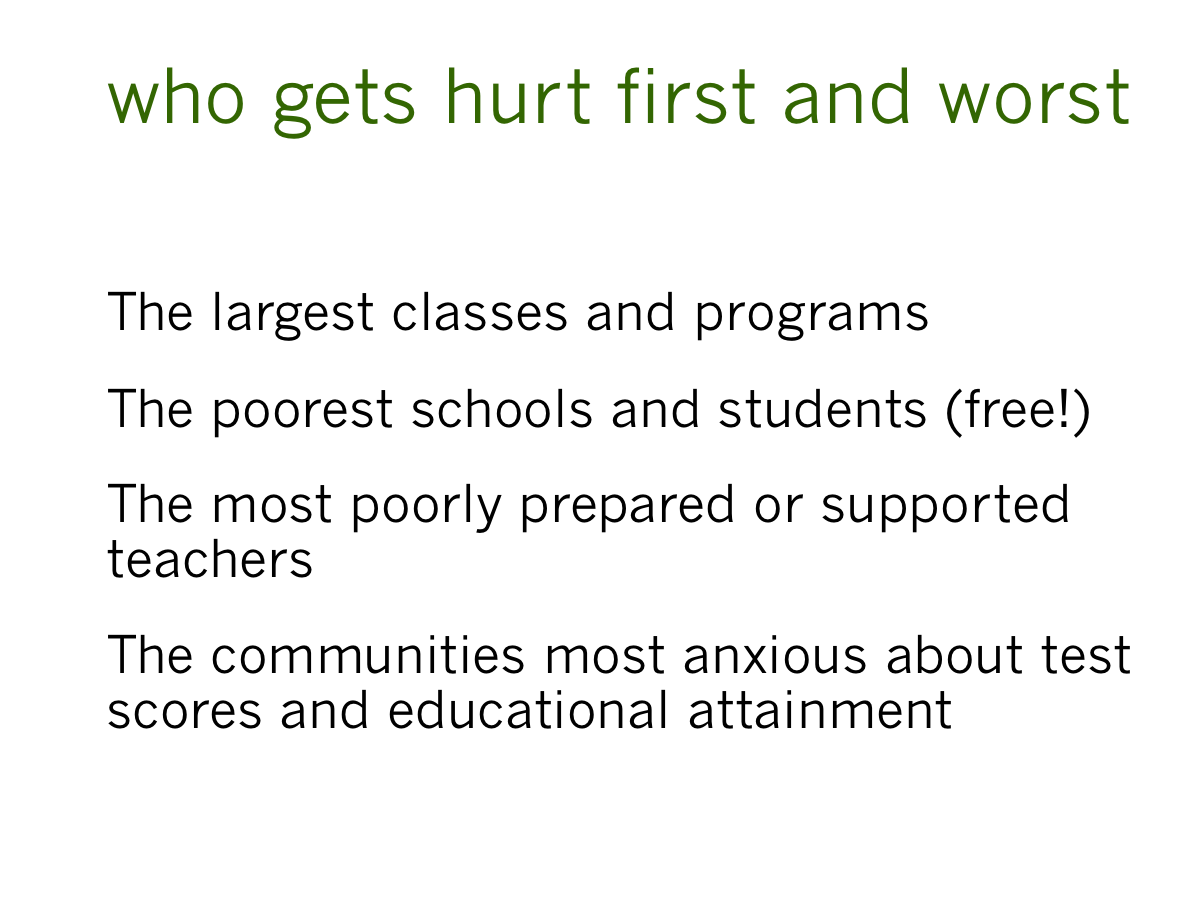
So who gets hurt? This is why I care so deeply. Who gets hurt first, and hurt worst? The largest classes and programs. The poorest schools and students. Those most poorly prepared and supported teachers. The communities most anxious about test scores and educational attainment.
Part 3: The Short History of Eli (version of why we started a company)
At this point, I want to put our own work on the table for inspection because when we started this part of our work (nearly a decade ago), we saw ourselves as part of this conversation.
In other words, what is Eli, where did it come from, and why did we start a company?
We started a business as the best way to change how writing is taught in the US.
To this audience, I may have just made a crazy claim. Which is another way of saying that it might seem an odd if not contradictory decision to this audience.
First, what is Eli and where did it come from? (editor’s note – for the full origin story, see the Eli Review site)
Now, why a company?
Once we created Eli, we had decisions to make: write about it, give it away, make it open source?
We are academics, and so by disposition and ideology, we are inclined to give away ideas and other forms of intellectual property. We publish, we share, and we are delighted when people listen, take up our ideas, and make them better. Our first impulse was to write about Eli, and we did to some extent and will continue to do so more frequently.
But there wasn’t—and still isn’t to this day—a community of writing teachers, researchers, and digital humanists willing and capable of supporting complex, long-term open source or similarly shared projects.
And we also know that there is no such thing as free, that the adoption of a pedagogy like Eli requires support.
In other words, the effective use of Eli requires a network of relationships to support it.
To sustain high quality software and high quality professional learning around that software requires resources that are predictable and reliable.
And that requires a sustainability model that led us to start, run, and maintain an organization that can make sustainable decisions.
Our argument is that if we provide a high quality *learning technology*—fundamentally a proven pedagogical scaffold + analytics—and the professional learning to support highly effective teaching and learning, then we will be a good partner for others.
And we measure ourselves based on our ability to help students and teachers learn—which is why this table make me cry a bit. Moving this needle is a bottom line measure for us.

Part 4: Engage
In this room and in our field we have lots of ways to think about technologies in relation to writing, just as we also have lots of ways to think about writing.
Tonight I am focusing our attention on technologies that support learning in writing in ways that are fairly commonplace, and I am absolutely insisting that this is where the most important action is right now.
- Important because it impacts many thousands if not millions of students.
- Important because the implications, both positive and negative, are highly stratified. Poor students, students in struggling schools, students whose first language is not English, and students whose community and home languages are not mainstream are being given bad robots.
- Important because the most commonly used technology to support learning in writing is TurnItIn—on your campus and on my campus.
- Important because so many of these robots understand learning in writing as a fundamentally individualized activity involving a student, a computer, and an algorithm.
Do we really think writing is best learned in isolation?
Via a narrow and empty notion of “personalized learning?”
Or do we think writing is one of the few things that makes us fundamentally human and that is learned in conversation with others?
If so, it’s time to engage.

It’s time to use our own pedagogies and technologies.
It’s time to make pedagogy in a way that is scalable and that will have oomph in the world.
It’s time to fix our gaze on the millions of learners who are being taught with technologies made by people who know very little about writing and learning to write.
It is one thing for us as researchers to say “My work is this” or “I am interested in that”
It is another thing entirely to say “The world needs me to solve this problem.”
The world needs you to solve some problems.
For more about how Jeff and the Eli Review team have written about how robots should support learning, see previous writing from:
Learn more about Eli Review, a pedagogy backed by technology:
- How it works
- Scaffolds student decision-making in giving feedback and using it to plan revision
- Positions instructors as
- designers who build reviews that help students give effective feedback
- coaches who debrief review and guide writers’ thinking between drafts
Reactions on Social Media
Jeff’s keynote address was delivered at the 2016 meeting of Computers & Writing, a crowd famous for its backchannel participation. We’ve pulled some of our favorite responses from the stream; if you want to see the full Twitter conversation, check out the stream.
https://twitter.com/PaulaMMiller/status/733791067062063104
https://twitter.com/PaulaMMiller/status/733800287283105792